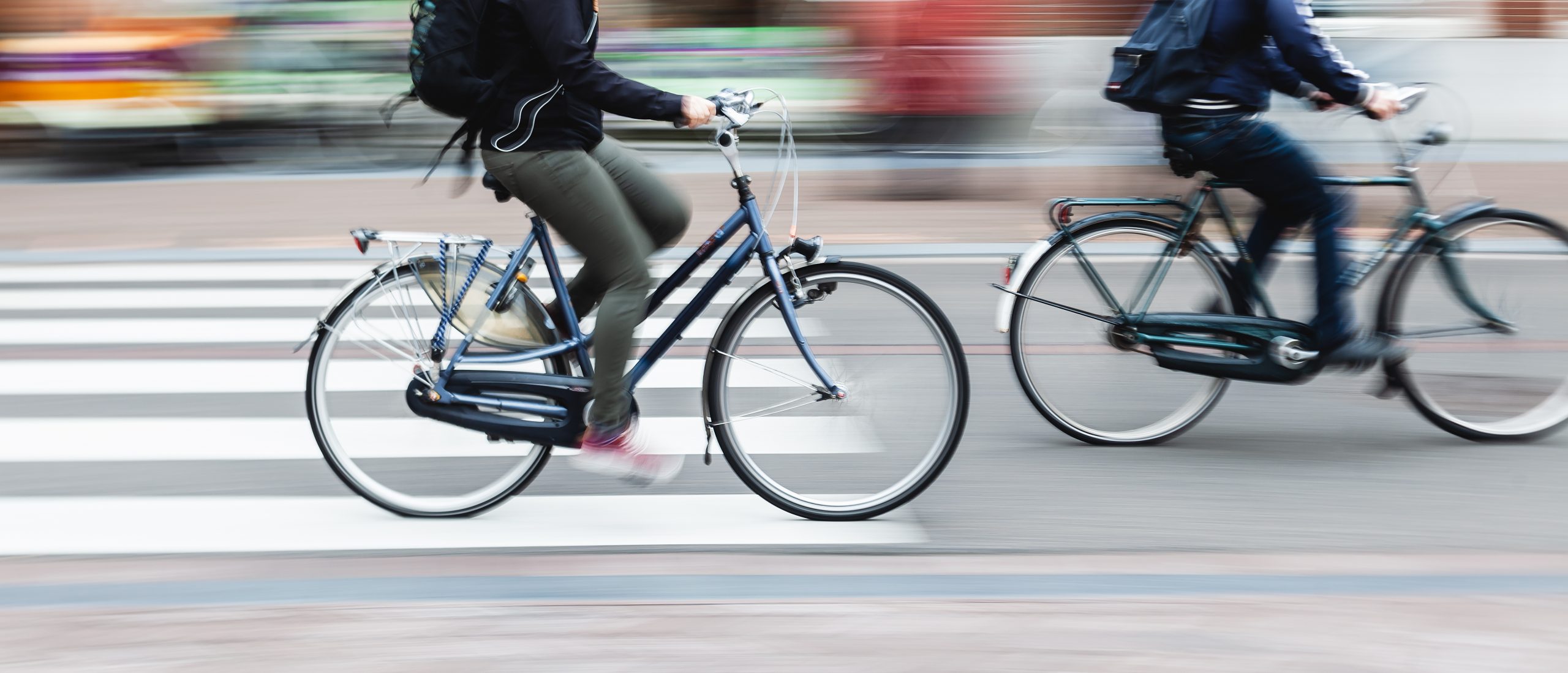
An unbiased look at traffic risks using smart sensors
Traffic accident statistics are proving resistant to attempts to reduce them. It is not easy to understand why: good data about traffic risks is scarce – and this gives rise to speculation and policies based on all too hasty conclusions. How can this data dearth be remedied? In their contribution, Michael Dubbeldam and Luuk van der Weide point to the possibilities of smart sensors.
Fat bikes have become hugely popular due to their speed, comfort, and – let’s be honest – their coolness. But this popularity comes at a price. There are now so many of them on our already-busy roads and bike lines, and they often travel at such speed, that many people are beginning to feel they are not safe in traffic. Media reports about the rise of fat bike-related accidents, often involving young victims, have only fed this perception.
The fat bike phenomenon clearly deserves closer attention. It is no surprise that doctors have recently teamed up with VeiligheidNL to register serious accidents involving fat bikes to obtain a clear picture of the problem. But the question is whether this emphasis on fat bikes isn’t unduly restricting our perspective on the problem of road safety. A 2008 study showed that young people in Amsterdam are cycling less often, and one of the reasons was busy traffic and the risks this involves. More than 40% of children in the first class of secondary school reported having fallen off their bikes at least once. And this was in 2008, long before the rise of the fat bike.
The question is whether this emphasis on fat bikes isn’t unduly restricting our perspective on the problem of road safety.
This raises the question how risky fat bikes actually are. And what about electric bikes and cargo bikes, which are often also deemed to be dangerous? What is the real impact of these modes of transport on road safety? Are there any other, underlying problems? And should we not at least include the possible advantages of these new forms of cycling in the equation? Maybe they allow older people to remain mobile for longer, encourage young people to exchange their mopeds or scooters for electric bikes, and motivate people with migration backgrounds to cycle more – wouldn’t that mean there are health advantages too?
There are, therefore, many good reasons to take a step back and look with an unbiased perspective at road safety risks. Unbiased in the sense of not ‘car-centric’, not supposing that everything was better in the good old days, and of having an open, honest and objective view. This requires scientific research, a certain remove from the topic and a healthy dose of skepticism, but above all data and facts.
And that’s the problem. Often all we have is data from accidents, hospital admissions, or citizen complaints – and this is too small a basis for good road safety analysis. What can we do to broaden this data basis?
Measuring road safety
One possibility is to include ‘near misses’ in the analysis, situations where an accident was narrowly avoided through intervention by a road user. These near misses normally occur much more often than actual accidents, and thus provide a fuller picture of road safety.
In fact, an interesting methodology for studying near misses already exists: the 1986 DOCTOR method: the Dutch Objective Conflict Technique for Operation and Research. This approach perfectly reflects the unbiased perspective we are looking for, but it does require some updating. It uses specially trained observers who classify and count near misses – an expensive solution that is not easily scalable. But what if we replaced the human observers by smart sensors?
This was the problem we tried to solve at the University of Twente and Technolution. Taking DOCTOR as our starting point, we used the smart traffic sensor FlowCube as our ‘eyes’, and ultimately succeeded in developing aa system that automatically detects near misses.
Machine learning
Our system’s smart sensor is an edge device, equipped with an image sensor and AI-optimized hardware. The ‘eye’ is positioned and calibrated in such a way that it monitors the traffic situation more or less from ‘above’. A multi-object tracking algorithm, in combination with a machine learning model, recognizes and classifies road users multiple times per second. The system uses this input to reconstruct an overhead view of the traffic situation on the site. By then connecting images from multiple sensors, we can very accurately determine speed, direction of travel, and position of road users on a street or bike lane. Indicators such as time to collision at current speed allow us to recognize near misses in the data – making road safety objective and measurable.
Indicators such as time to collision at current speed allow us to recognize near misses in the data – making road safety objective and measurable.
Infrastructure and privacy
This sounds high tech, and it is. And yet the sensor system is easy to install: it can be attached to existing infrastructure such as street lights or traffic lights. The mobile network is used for communication, so no specific adjustments are required for that either. This makes the installation feasible and affordable.
Privacy is an important concern, of course. Because this is an edge device, all processing of images takes place on the spot, which is demonstrably privacy friendly. Naturally, certain situations – the near misses – will have to be stored for evaluation purposes, but this happens in the form of low-resolution images, which contain enough information to study the safety situation but do not show any identifiable personal details.
Lessons learned
We have used the new sensor system in three trial projects. They have taught us that near misses can be detected and classified in five steps.
Step 1 involves detecting the objects. Step 2 consists of estimating the position of the objects detected in the public space. If there are multiple sensors on site, we can connect the data from each individual sensor to increase the accuracy of the positioning – this is step 3. In step 4, we estimate the dimensions of the objects, which gives us a 3D image of the traffic situation. This allows us in step 5 to detect and classify near misses: was it a bike-car incident, a van-car incident, a bike-bike incident, etc.?
The system creates an animation (overhead view) of every incident detected, which shows precisely what happened. The sensor is not a black box, but a transparent and verifiable aid to assess and evaluate road safety risks.
Unbiased
Just to return to the problem of the fat bikes. How could we use this sensor-based approach to look without bias at the risks of this mode of transport?
Thanks to machine learning, the system can recognize and classify objects. Passenger vehicles, public transport buses, school buses, freight trucks, motorbikes, cyclists and pedestrians are all clearly recognized, but, due to their distinctive physical traits, so are fat bikes and cargo bikes. This means it is easy to compile statistics about speed, numbers, and routes of the various modes of transport.
Targeted monitoring of traffic situations that include fat bikes will therefore provide interesting and fact-based insights. How do road users behave? At what speeds do they travel? Where and how often do near misses occur? Or, if measures have been taken such as banning soup-up kits: how effective are they?
Our open perspective will be further enhanced because near misses that have nothing to do with fat bikes will also be ‘seen’. This makes it possible to assess how the risks associated with fat bikes compare to the risks associated with cars, scooters, cargo bikes, ordinary bikes, and crossing pedestrians. Fat bikes will thus no longer be recognized only as the cause of dangerous situations but also as actors who are involved unintentionally in such situations.
Another interesting aspect is that we can monitor traffic flows by combining data from multiple intersections. This provides valuable information on traffic density, directions of travel, and bottlenecks: the context in which near misses occur. No longer will we have to go on suspicions and annoyances; we can now stick without bias to the facts.
The authors
Michael Dubbeldam is a Senior Architect at Technolution and is in charge of Vision AI.
Luuk van der Weide is a Machine Learning Engineer at Technolution. He was affiliated with the University of Twente at the time of the study.